Current research thrusts at the Signal Processing and Information Systems (SPIS) Laboratory span a number of domains including inverse problems and computed imaging; sparse signal representation; signal and pattern analysis; and data fusion. Please see below for brief information on a subset of specific recent research efforts. |
Characterizing Neural correlates of Emotional Word Processing from English Words
|
|
Studying emotional responses to words as the fundamental blocks of verbal and auditory messages has diverse applications in model development for cognition and psychology theories, linguistic studies, semantic analysis, neuromarketing, and the like. These fields usually consider two-dimensional or three-dimensional theories of emotion processing which assign features of valence, arousal, and dominance for emotional load of every word in a language. As such, recording and characterizing subjective responses to the emotional load of words, whether used in isolation or together with accompanying words in a specific context, have interesting implications in understanding personal interactions with emotional messages as well as intensifying emotional experience in communication settings. In this study, behavioral responses and EEG recordings of native and bilingual speakers while reading emotional texts in two different languages are collected and characterized.
|
Adapting Brain-Computer Interfaces to Users’ Cognitive States
|
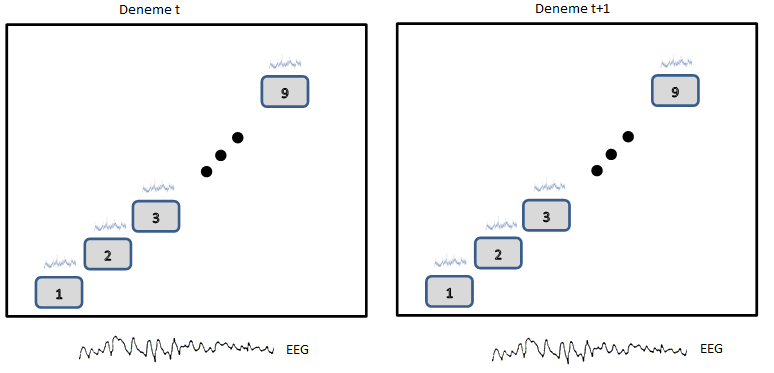 |
With increasing demands for daily and long-term use of BCIs, these systems have been tested on longer sessions in which lower performance has been observed. Nonstationarity of the brain rhythms is believed to play an important role here and is, in some extents, affected by changes in the cognitive states of the user such as cognitive load, attention, fatigue, and motivation. Dynamically extracting information about such cognitive states from EEG data and using it to improve the performance of BCI systems by taking advantage of combined active and passive BCIs are the current goals of this research. The data collection phase of this study is complete and the analysis phase using advanced signal processing and machine learning schemes is being carried out. |
Statistical Methods for a Fine-Grained Retail Product Recognition System
|
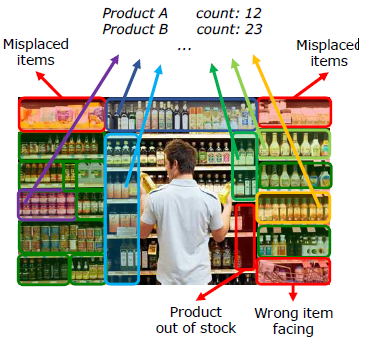 |
In the past few years, product recognition applications have gained increasing interest in computer vision. Retail product classification system can be used for assisted shopping by the customers, tracking of the consumer product arrangements on the shelves, and real-time management of inventory distortions such as out-of stock and overstock. Fine grained classification is one of the challenging problems in computer vision. In retail stores, there are a large number of fine-grained product classes and many products have similar appearance in terms of shape, color, texture and metric size. Besides, the product images are captured under real world conditions. So, the captured images are very likely to suffer from many problems such as different viewing angles, blurriness, occlusions, unexpected background parts, and very different lighting conditions. Such complications in the images make the retail product recognition problem more challenging. Accordingly, an effective product classification system needs further information in addition to knowledge obtained from the product image. This project aims for developing statistical and machine-learning based methods for a fine-grained retail product recognition system. |
Moving Object Imaging from Imaging Radar Data
|
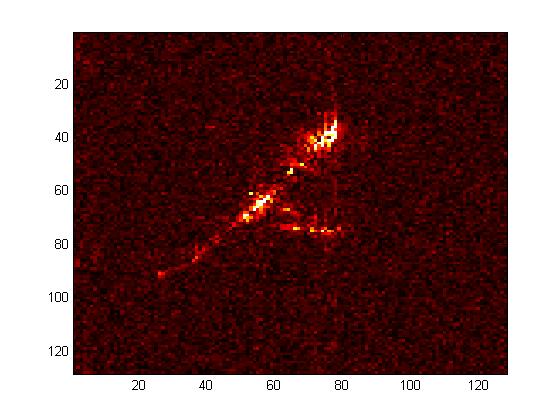 |
The data of moving targets acquired by imaging radars such as synthetic aperture radar and inverse synthetic aperture radar can not be properly focused using methods developed for stationary targets. To carry out focusing of moving targets, we are developing methods based on sparse reconstruction principles, which can generate high-resolution images from data undergoing realistic motion. The methods being developed can also work if the data have missing samples. |
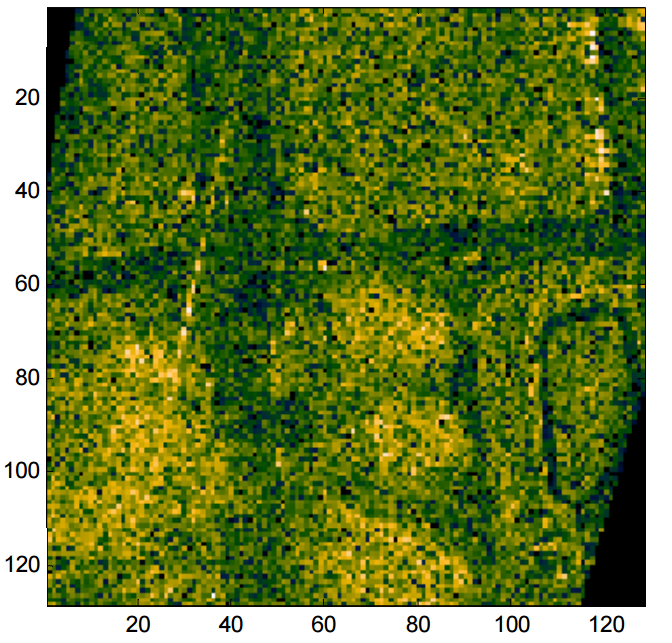 |
An important aspect of moving target imaging with SAR is to extract the moving targets from the stationary background. These extracted targets can be focused subsequently with various focusing algorithms. We are working on Low Rank and Sparse Decomposition (LRSD) based approaches for target extraction. |
Spelling through a P300-based Brain-Computer Interface
|
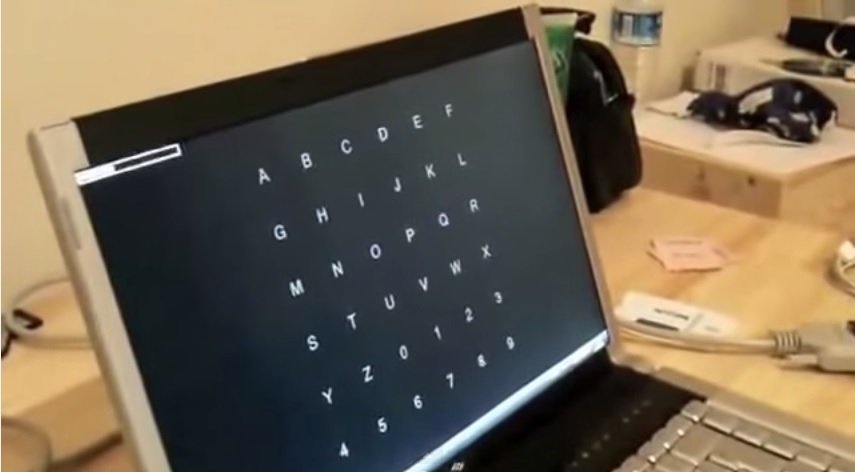 |
This is the fastest implementation of a P300 speller system ever published in an academic work up to 2010, and has been published in various conference proceedings by Armagan Amcalar and Mujdat Cetin. |
|
|
Dendritic Spine Analysis
|
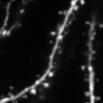 |
Dendritic spines, membranous protrusions of neurons, are one of the few prominent characteristics of neurons. Neuron activity changes modify spine morphology. Spine morphological analysis can play a significant role in inferring the inherent relationship between neuronal activity and spine morphology variations. This analysis is currently performed manually due to the deficiency of fully automated and reliable tools. This project aims for developing new probabilistic and machine-learning based image processing algorithms for the dendritic spines classification from two-photon microscopy images. |
|
|
Model Error Correction in SAR Imaging
|
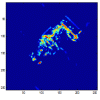 |
We are working on a framework, in which image formation and model error correction in SAR imaging are jointly evaluated. |
|
|
Development of Electroencephalography (EEG) Signal Analysis Techniques for Brain Computer Interface (BCI) Systems
|
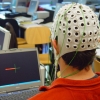 |
Electroencephalography (EEG) based Brain-Computer Interface (BCI) systems are a new development in the field of applied neurophysiology. In this project, our objective is to develop new statistically-driven signal analysis and pattern recognition algorithms for BCIs and to demonstrate the effectiveness of these algorithms both on standard data sets and on the data collection and BCI system we have set up. |
|
|
Communication Constrained Distributed Estimation
|
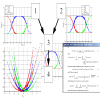 |
We conduct research along subjects related to Collaborative Signal and Information Processing motivated by networked sensing. |
|
|
Coupled Non-Parametric Shape and Moment-Based Inter-Shape Pose Priors for Multiple Basal Ganglia Structure Segmentation
|
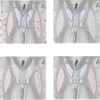 |
Brain tissue and structure segmentation in magnetic resonance (MR) images is a fundamental problem in clinical studies of brain structure and function. Due to limitations such as low contrast, partial volume effects, and ?eld inhomogeneities, the delineation of subcortical (basal ganglia) structures such as caudate nucleus, putamen, and thalamus from white matter, gray matter and cerebrospinal fluid (CSF) is a very challenging problem. |
|
|
Regularized Image Reconstruction
|
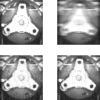 |
Our research is mostly concentrated on the selection of the regularization parameter when a non-quadratic regularizer is incorporated to the solution. |
|
|
Machine Learning Systems For Detecting Driver Drowsiness
|
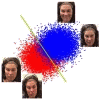 |
In this project the development of machine learning systems for drowsy driver detection is proposed. |
|
|
Stereo Based 3D Head Pose Tracking
|
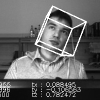 |
In this project a new stereo-based 3D head tracking technique, based on scale-invariant feature transform (SIFT) features, that is robust to illumination changes is proposed. |
|
|
Graphical Model Based Facial Feature Point Tracking
|
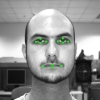 |
Feature point tracking is a challenging topic in case of arbitrary head movements and uncertain data because of noise and/or occlusions. With this motivation, a graphical model that incorporates not only temporal information about feature point movements, but also information about the spatial relationships between such points is built.
Related videos:
|
|
|